Optimizing Your Kubernetes Resources with Helm
On-Demand FinOps Training
Automated K8s Cost Management with Helm
If you’re part of a team managing a containerized environment using an orchestration technology like Kubernetes (K8s), then you know the pains of having to write and maintain YAML manifests: Duplicated values, hardcoded transient variables—even when app developers are involved with managing the environment, the situation is a nightmare.
Helm to the rescue! But, what about when developers either forget to include appropriate resource specifications or they request unnecessary resources for their applications? We all know where that leads: low infrastructure utilization, more nodes, and out-of-control costs—and more work for you.
Watch this training and learn how Helm can be used to automatically configure the optimal resource specification for each container, whenever an application is installed or upgraded. The demo will reveal how cost and time savings can be gained through more precise, automated configuration of container resources.
Automated Kubernetes Cost Optimization
About the Presenters
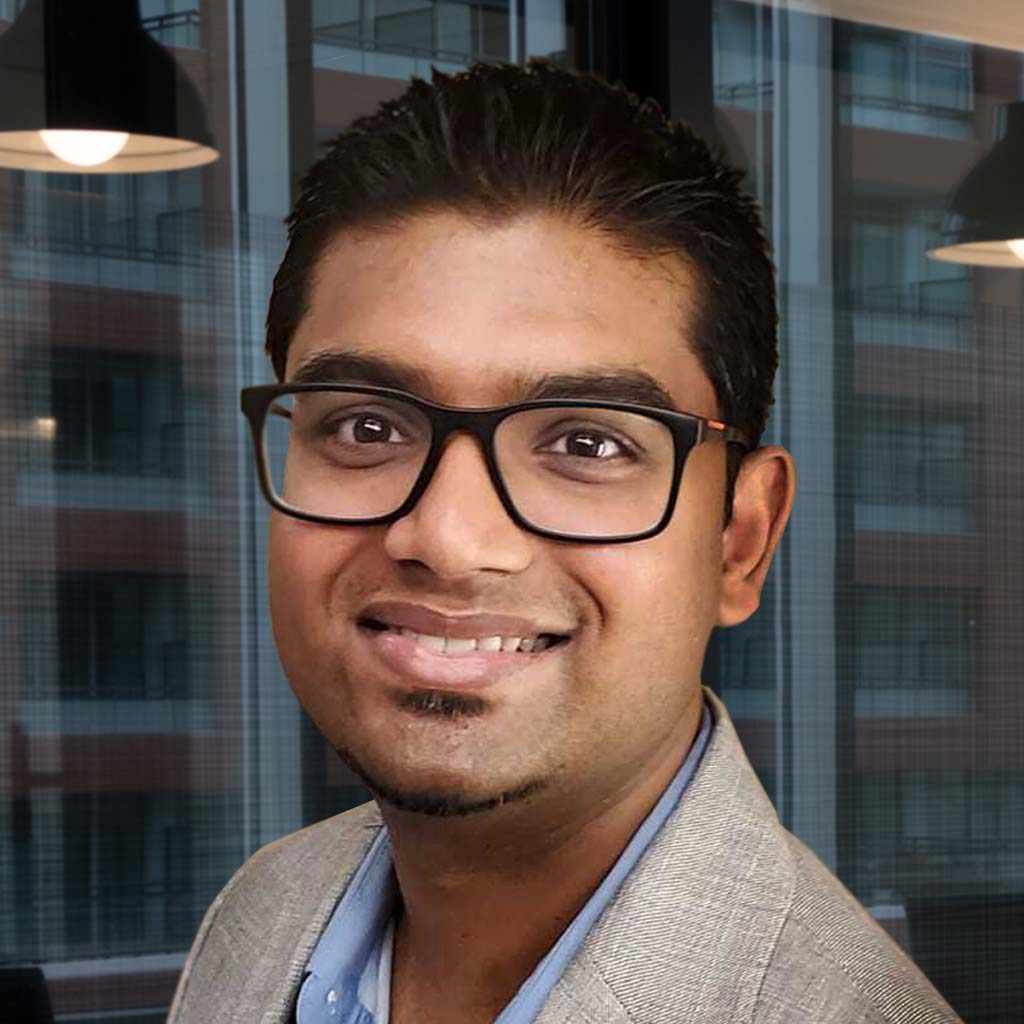
Dinesh Raveendran Cloud Architect, Densify
Dinesh Raveendran has 15 years of experience owning, managing, and delivering cutting-edge technology propositions. He is responsible for explorative research into existing and emerging cloud management technologies, and evangelizing how these technologies can be leveraged to help optimally manage cloud infrastructures through prototyping, thought leadership, and content creation.
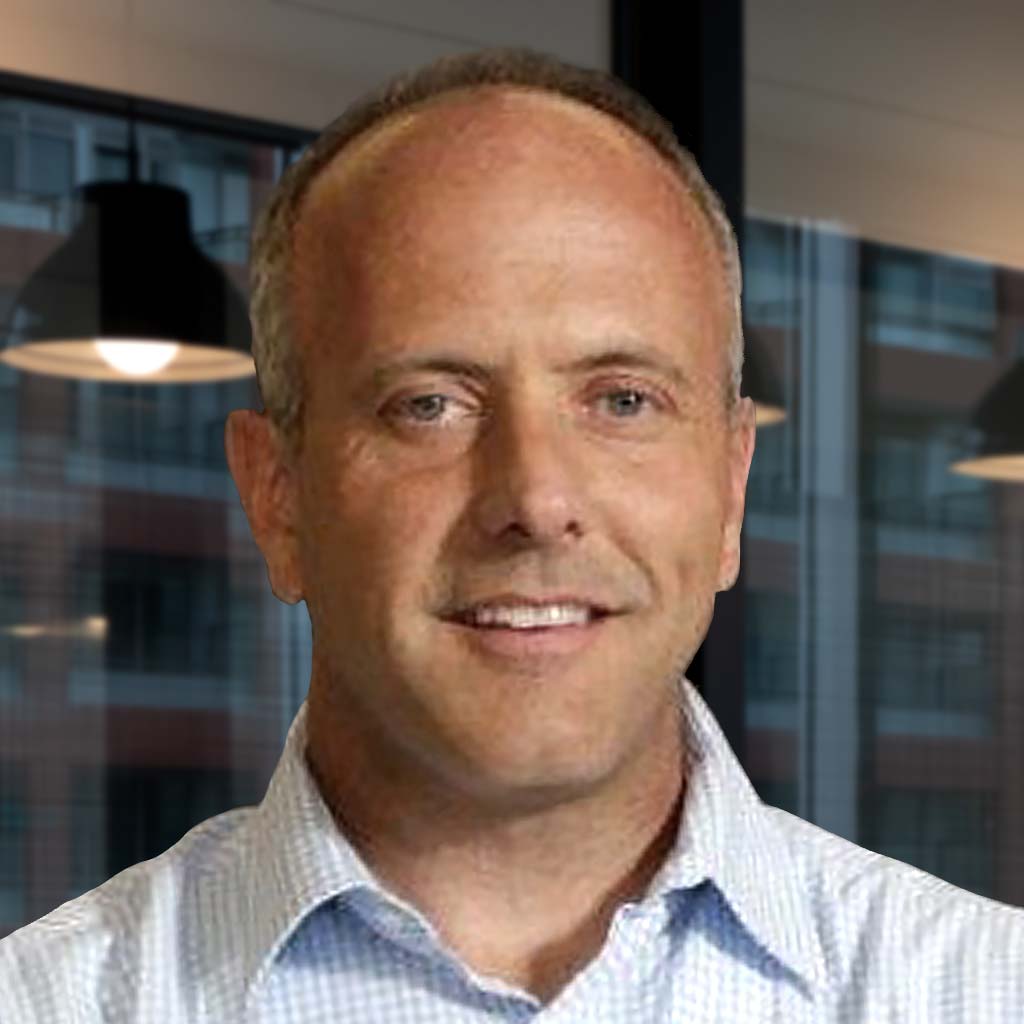
Chuck Tatham Chief Marketing Officer & VP, Business Development, Densify
Chuck Tatham has 20 years of experience in enterprise software marketing, sales, and corporate management. As CMO and VP Business Development, he is responsible for global marketing and strategic business development initiatives.
Prior to Densify, Chuck held management roles at Compuware Corporation, Changepoint Corporation, and Lotus Development. He holds a Bachelor of Science degree in psychology from The University of Toronto.